9 HR Analytics Terms Every HR Professional Must Know
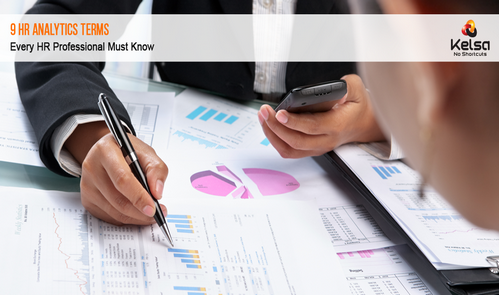
People Analytics, also known as HR analytics has garnered a lot of interest from business leaders for the in-depth insights it can offer on workforce attitudes, preferences and behaviour as well as efficacy within organisational team structures and workflows. The insights they deliver enable clarity and ensure improved quality in organisational decision making.
To many of us, people analytics and related tools seem fascinating and versatile, yet they are riddled with vague and unfamiliar sounding terms. So, let’s begin with exploring the popular terms used in analytics, and next, we will examine how companies can use these to drive process efficacy, engage employees better and grow and transform work environments.
Here are the top 9 analytics tools and terms that HR data analysts and data scientists would typically refer to while steering your people analytics process.
Data mining
Sifting through tons of data to explore information and discover the patterns within. It helps create tangible/ predictive information and aids decision making and process planning to improve outcomes.
Machine learning
Techniques through which a computer becomes familiar with the data provided, and learns to analyse and identify patterns within.
Structured and unstructured data
Structured data are well organised and managed within defined formats such as an MS Excel spreadsheet or database – for example, HR records with employee name, joining information, education and work background details etc. Unstructured data refers to information spread across multiple formats (e-mails, group circulars and communications) and which need processing to extract meaningful information.
Supervised and unsupervised learning
Supervised learning is enabled with output data being made available or defined to enable emerging information patterns to be easily decoded. With unsupervised learning however, no output data is provided and patterns and predictions are synthesised by clustering sets of related points within the data.
Clustering
It is a kind of machine learning capability where predictions are achieved through clustering data and generating an estimation through observed similarities.
Training data and test data
These are two distinctive data sets generated by splitting a uniform and common body of data, and aid with testing the accuracy of predictive algorithms.
Overfitting
The tendency to overfit algorithms to conform with desired ideas and understanding – a real risk in the area of data sciences, which analysts work hard to eliminate.
R
An open-source system, that can be accessed as a free tool, and offers robust capabilities in statistical computation, prediction and visualization.
Decision tree
Decision tree models enable understanding on decisions and possible outcomes, and help with making quality predictions.
Having established a fair understanding of the commonly used tools in delivering people analytics, let’s examine how people analytics initiatives are being driven within companies and the benefits it helps achieve.
CASE STUDY – Decoding key traits/ drivers for sales performance within a large financial services company
A large American financial services company had long hired sales staff mainly from a pool of top performers from highly ranked colleges. Academic performance was seen as key evidence in predicting on-the-job performance and organisational fit, and all recruitment and selection processes were anchored to academic drivers.
However, a detailed analytics exercise that compared and synthesised sales productivity and employee turnover data over the first two years of an employees’ tenure, presented some interesting results. For instance, data patterns revealed that key predictors for sales productivity at the time of hiring include –
- A well presented resume that is accurate and grammatically correct.
- Continued education from school through college without any breaks/gaps.
- Successful sales experience in previous jobs, especially when within real estate and automotive industries.
- Comfort with ambiguity and unstructured conditions.
Barring the above, none of the other factors that had been previously considered such as academic grades, highly ranked colleges and high quality references seemed relevant to predicting the new employee’s sales performance.
By actively listening to the patterns emerging from the data, the company stepped away from a a 30 year belief system and adopted a newer approach to recruitment. The change in strategy did pay off handsomely and helped the company to drive a $4M improvement in sales revenues within the next fiscal period.
People Analytics and Data Discipline
Companies today are loaded with data that help them understand key events and success factors through the employee lifecycle. With most of them using automated tools to record, store and manage employee and performance information, mining and extracting relevant data is easy.
And people analytics tools are easily deployed within such environments to drive quality decision making and several high-return applications including efficacy in recruitment, employee engagement and retention, benefits adminsitration, and developing success factors for sales performance, leadership pipeline and development.
The key to ensuring successful deployment of these high return applications though is knowing how to maintain and monitor data with discipline and manage it scientifically to drive people related decisions.
Data analytics involves real discipline and demands skill across several areas including cleaning, defining statistics, visualization, and problem-solving. These are skill areas that most HR professionals are still unfamilar with; companies need to find ways to ensure a culture that values data backed evidence and commit to bring together people and ideas to leverage and develop on HR data.
9 HR Analytics Terms Every HR Professional Must Know
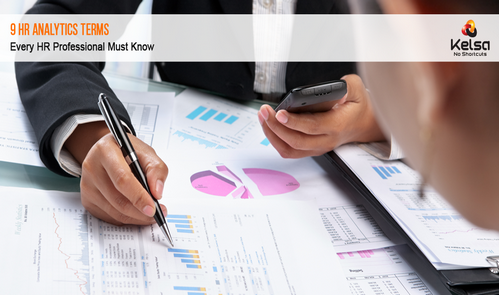
People Analytics, also known as HR analytics has garnered a lot of interest from business leaders for the in-depth insights it can offer on workforce attitudes, preferences and behaviour as well as efficacy within organisational team structures and workflows. The insights they deliver enable clarity and ensure improved quality in organisational decision making.
To many of us, people analytics and related tools seem fascinating and versatile, yet they are riddled with vague and unfamiliar sounding terms. So, let’s begin with exploring the popular terms used in analytics, and next, we will examine how companies can use these to drive process efficacy, engage employees better and grow and transform work environments.
Here are the top 9 analytics tools and terms that HR data analysts and data scientists would typically refer to while steering your people analytics process.
Data mining
Sifting through tons of data to explore information and discover the patterns within. It helps create tangible/ predictive information and aids decision making and process planning to improve outcomes.
Machine learning
Techniques through which a computer becomes familiar with the data provided, and learns to analyse and identify patterns within.
Structured and unstructured data
Structured data are well organised and managed within defined formats such as an MS Excel spreadsheet or database – for example, HR records with employee name, joining information, education and work background details etc. Unstructured data refers to information spread across multiple formats (e-mails, group circulars and communications) and which need processing to extract meaningful information.
Supervised and unsupervised learning
Supervised learning is enabled with output data being made available or defined to enable emerging information patterns to be easily decoded. With unsupervised learning however, no output data is provided and patterns and predictions are synthesised by clustering sets of related points within the data.
Clustering
It is a kind of machine learning capability where predictions are achieved through clustering data and generating an estimation through observed similarities.
Training data and test data
These are two distinctive data sets generated by splitting a uniform and common body of data, and aid with testing the accuracy of predictive algorithms.
Overfitting
The tendency to overfit algorithms to conform with desired ideas and understanding – a real risk in the area of data sciences, which analysts work hard to eliminate.
R
An open-source system, that can be accessed as a free tool, and offers robust capabilities in statistical computation, prediction and visualization.
Decision tree
Decision tree models enable understanding on decisions and possible outcomes, and help with making quality predictions.
Having established a fair understanding of the commonly used tools in delivering people analytics, let’s examine how people analytics initiatives are being driven within companies and the benefits it helps achieve.
CASE STUDY – Decoding key traits/ drivers for sales performance within a large financial services company
A large American financial services company had long hired sales staff mainly from a pool of top performers from highly ranked colleges. Academic performance was seen as key evidence in predicting on-the-job performance and organisational fit, and all recruitment and selection processes were anchored to academic drivers.
However, a detailed analytics exercise that compared and synthesised sales productivity and employee turnover data over the first two years of an employees’ tenure, presented some interesting results. For instance, data patterns revealed that key predictors for sales productivity at the time of hiring include –
- A well presented resume that is accurate and grammatically correct.
- Continued education from school through college without any breaks/gaps.
- Successful sales experience in previous jobs, especially when within real estate and automotive industries.
- Comfort with ambiguity and unstructured conditions.
Barring the above, none of the other factors that had been previously considered such as academic grades, highly ranked colleges and high quality references seemed relevant to predicting the new employee’s sales performance.
By actively listening to the patterns emerging from the data, the company stepped away from a a 30 year belief system and adopted a newer approach to recruitment. The change in strategy did pay off handsomely and helped the company to drive a $4M improvement in sales revenues within the next fiscal period.
People Analytics and Data Discipline
Companies today are loaded with data that help them understand key events and success factors through the employee lifecycle. With most of them using automated tools to record, store and manage employee and performance information, mining and extracting relevant data is easy.
And people analytics tools are easily deployed within such environments to drive quality decision making and several high-return applications including efficacy in recruitment, employee engagement and retention, benefits adminsitration, and developing success factors for sales performance, leadership pipeline and development.
The key to ensuring successful deployment of these high return applications though is knowing how to maintain and monitor data with discipline and manage it scientifically to drive people related decisions.
Data analytics involves real discipline and demands skill across several areas including cleaning, defining statistics, visualization, and problem-solving. These are skill areas that most HR professionals are still unfamilar with; companies need to find ways to ensure a culture that values data backed evidence and commit to bring together people and ideas to leverage and develop on HR data.